So, last week I had a calculus assignment, and guys, it was not easy for me to resolve it using my timid mind. After hours of sitting idle and worrying about how to complete that assignment, a friend suggested I try my hands on the mastermind, AKA computer. But do you think it is that simple?
It will need a GPU that works for deep learning to have your computer do all the calculus stuff for you. We all hear GPUs are designed specifically for graphics processing, playing high-end games, and editing. However, a savvy tech friend of mine told me that you can actually use a GPU for more than just graphics.
Like you can use them to process scientific data, calculate the optimal route on a map, or do any other calculations that would take longer if done manually. Worry not, as I will share all those handy points that helped me get the best GPU for deep learning, along with 5 of the best picks I have tried and tested. So, keep on reading!
Top Products:
Best GPU For Deep Learning Reviewed At a Glance:
Best GPU For Deep Learning Reviewed
NVIDIA Titan V
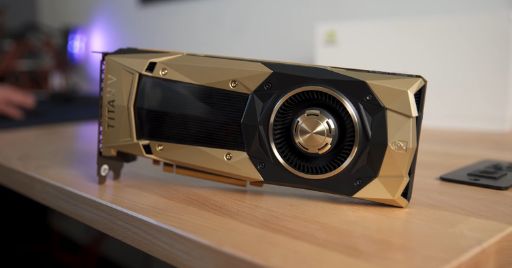
Powerful GPU For Deep Learning
SPECIFICATIONS
Brand: NVidia | Graphics Coprocessor: NVidia Titan | Graphics Manufacturer: NVidia | RAM Size: 12 GB | Clock Speed: 1700 MHz
And guys, when I tell you that the NVidia Titan V is one of the most expensive GPUs on the market, trust me, I am not kidding. And, of course, we can expect a premium price tag for these looks. Golden aesthetics and that metallic shine it does give that luxe visual; let’s get to see whether it can deliver the premium performance or not.
From visuals, I got to see a single cooling system, which is the same one on the NVidia XP. Eventually, the heatsink was the same as well, so I went on to explore both of them. Now, before you purchase this one for gaming, let me stop you here. The NVidia Volta, without any doubt, is a powerful GPU for deep learning.
Still, it won’t give the same performance while playing games. This might be a massive disappointment for gamers, but it is what it is! So, first, I will be looking at the frame rate NVidia V has; honestly, I found the frame rate a bit boosted up in Cuda cores compared to the previous NVidia XP.
Also, I learned that the core clock is limited, but it happens when you increase the core count. Another feature that I came across on Titan V is that it comes with increased tensor cores. Because of these, it worked wonders for deep learning activities.
Okay, I found that this card has a peak frequency of 1682 MHz for overclocking abilities. Honestly, this card has spacious overclocking headroom, but you will need to liquid cool it for it to run cool. Next, I checked the boost functions and found the same ones with the NVidia Pascal architecture GPU.
For getting benchmarks, I tried some games, which gave me 12308 points for the Titan V. Here, I found that the results were about 22% ahead of the Titan XP. Also, the Titan V points were close to the MSI 1080 ti when overclocked.
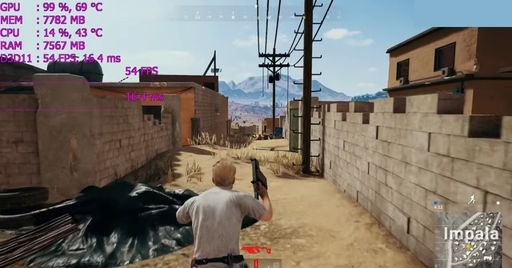
From the results, it was evident that Volta actually leads the graphics test at 23%. Next, I tried using the NVidia V for testing tessellation, which usually involves geometry and other calculations. So, the results showed that Volta had around 20 to 21% lead in the graphics card test 2.
REASONS TO BUY
✓Powerful Card
✓Works best for deep learning
✓Runs at 24 fps
✓Solid build
REASONS TO AVOID
✗Expensive
WHY YOU SHOULD CHOOSE THIS GPU?
Honestly, if you are willing to spend $3000 on a GPU, then the NVIDIA TITAN V is the world’s most powerful GPU for deep learning. I am in awe of its solid build and powerful performance.
NVIDIA Titan RTX
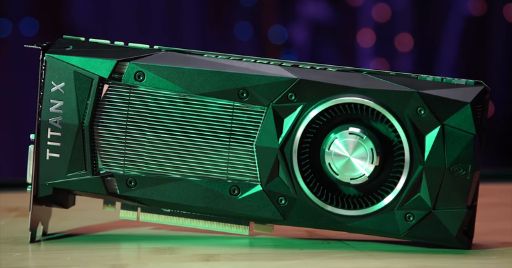
Overall Best GPU For Deep Learning
SPECIFICATIONS
Brand: NVidia | Graphics Coprocessor: NVidia Titan X Pascal | Graphics Manufacturer: NVidia | RAM Size: 12 GB | RAM Type: GDDR5 | Clock Speed: 11.4 GHz
As of today, I am testing all the best GPU for deep learning, so all the picks here are best for that purpose only. The NVidia Titan XP is the best GPU for deep learning; however, I won’t recommend it for gaming.
The XP is best for things like neural-net or machine learning applications. Here the architecture is the pascal, and because of this, they are known as XP. At the same time, the previous one had a Maxwell architecture known as NVidia Titan X.
In terms of connectivity with this one, you are getting an HDMI 2.0 port and one dual-link DVI i3. With such specs, I could output an 8K 60-hertz signal for a multi 4K setup. Also, it comes with SLI connectors that support high bandwidth SLI bridges. Further, it comes with one eight-pin and one six-pin power connector.
Now the Titan X runs at a lower boost; the XP had made up for that by having a whopping 3584 Cuda Cores. And in general, guys, this is much greater than 1080. So, you already know that this GPU supports a GP 102 graphics processor; some of these Cuda cores are not working with the XP.
If you want all of these cores working, you can get a Quadro P6000. But I won’t suggest you go for that until and unless you are working on rendering videos. I first tried playing games over this one to get the benchmarks, so the results showed that the Titan XP can beat 1080 by about 10 FPs.
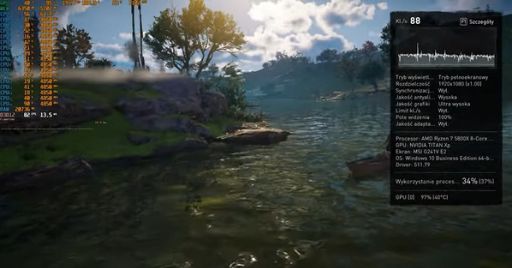
But then you can get two 1080s for the price you will be paying for the Titan XP. Now let’s talk about the frame rates. So I wasn’t entirely satisfied with this frame rate; however, everyone would expect a lot because it comes with the name Titan.
But the low frame rates proved that the titan XP is only suitable for processing and not gaming. I would suggest going for the 1080ti or some other GPU but not this one for gaming.
REASONS TO BUY
✓Fastest GPU
✓Plenty ports
✓Handles algorithms easily
✓Let’s you play games
REASONS TO AVOID
✗Expensive
WHY YOU SHOULD CHOOSE THIS GPU?
With 12GB of GDDR5 memory, this NVidia Titan RTX graphics card offers the same level of performance as a top-of-the-line RTX 2080, with up to six times the capacity and up to twice the power efficiency. However, I suggest you only go for this one if you are into processing algorithms and not gaming.
NVIDIA GeForce RTX 2080 Ti
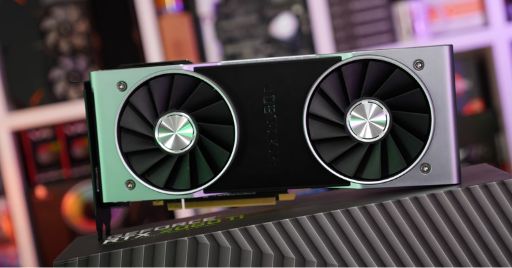
Best GPU For Deep Learning
SPECIFICATIONS
Brand: NVidia | Graphics Coprocessor: NVIDIA GeForce RTX 2080 Ti | Graphics Manufacturer: NVidia | RAM Size: 11 GB | RAM Type: GDDR6 | Clock Speed: 14000 MHz
The NVidia GeForce RTX 2080 Ti is the best GPU for deep learning. I would definitely get it again and again for my system for deep understanding. Let’s first compare it to the previous GPU RTX 2080. So, 2080 has 46 RT cores, while 2080 ti has 68 RT cores.
Regarding the RTX-OPs, 2080 has 57 references and 76 references. Lastly, the Giga rays on 2080 are 8, and the 2080 ti is 10. Additionally, the tensor cores on 2080 are 368, while on the 2080 Ti, it’s 544, so you can see who is leading here, of course, the RTX 2080 Ti. And these tensor cores will help you for deep learning anti-aliasing purposes.
Also, using the DLSS with ultra-high quality, this GPU can achieve 64X super sampling. Now let’s have a look at some of the gaming benchmarks. First, I tried playing Far Cry 5 over this one at 4K settings.
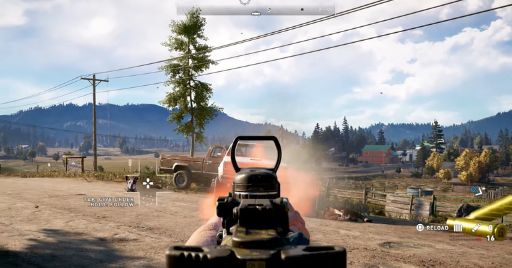
The 2080 Ti can deliver some good results in the 70s, and I feel relieved here because, again, this was not the GPU for gaming; still, it performed well.
I also tried the LuxMark tests and found that the 2080 Ti performs similarly to the Titan V, which is a much more expensive card.
The manufacturing process for the 2080 and 2080 Ti has shrunk from 16 NM to 12 Nm; still, the latter is a bit more costly than the former one.
REASONS TO BUY
✓Easiest to install
✓Rock-solid quality
✓Best for deep learning
✓Best value GPU for the price
REASONS TO AVOID
✗Limited drivers
WHY YOU SHOULD CHOOSE THIS GPU?
The NVidia GeForce RTX 2080 Ti is the best GPU for Deep Learning. It has the highest amount of VRAM for the size of its memory. It has the best performance at half the cost, so if you want a Titan V performance at a much decent price, then get yourself a 2080 Ti.
NVIDIA TeslaV100
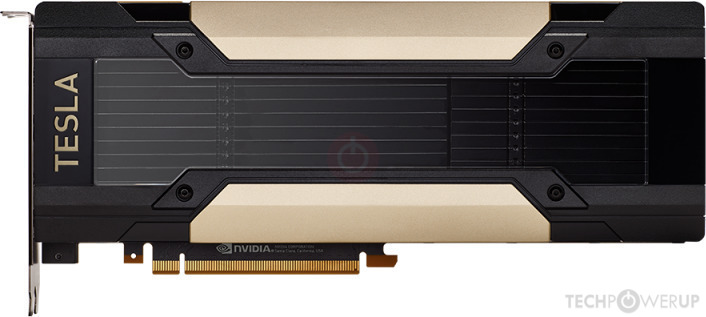
Top Rated GPU For Deep Learning
SPECIFICATIONS
Brand: PNY | Graphics Coprocessor: NVidia Tesla v100 | Graphics Manufacturer: NVidia | RAM Size: 16 GB
Now, if you have to ask me about this new GPU Tesla V100, trust me, it is not less than a luxury GPU available in the market. Guys, this beast is literally a Bugatti Veyron, yes, you read that right, and you are just thinking right; it is one of the fastest cars in the world.
And with that said so, guys, it is costly; you would definitely have to break your bank account just to grab one of these cars; unless you are a millionaire, you can have two of them. Plus, maintaining this car is impossible because it would cost you a lot of fortune.
The same is the case with V100. So, what makes this GPU so unique, and why is it the most hyped GPU for deep learning in the market? Now there are a lot of reasons for that. After borrowing one from my friend, I tested the V100 for many computational purposes.
The first one was the Computational Fluid dynamics and some rendering that I tried my hands on. Okay, guys, I wanted to ensure the V100 has an FP64 computation, and this one had some high numerical precision.
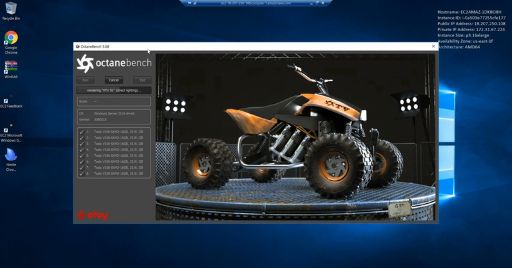
Now the next thing I liked about this GPU was a whopping 32 GB of storage; this makes it the fastest GPU for deep learning. If I talk about the 2080 Ti, I would say that it will give you the same performance as the V100 but at a lower price than what you will pay for the V100.
Now, I would not recommend using this for gaming or any other recreational purposes because the architecture is not designed to do so. I will only have this if I work over deep learning; otherwise, you must stir off this. So, if you have saved money burning up in your pocket, this can be the right purchase.
REASONS TO BUY
✓Maximum deep learning performance
✓A large amount of memory for machine learning
✓Top-notch AI performance
✓Fastest running GPU
REASONS TO AVOID
✗None
WHY YOU SHOULD CHOOSE THIS GPU?
Overall I am in love with the NVidia Tesla V100. However, I won’t suggest this one for gaming because it can cost you a lot and still won’t deliver the right performance. Besides that, the memory is excellent, and you will be able to store lots of data. The AI performance is also impressive.
NVIDIA GeForce RTX 2070
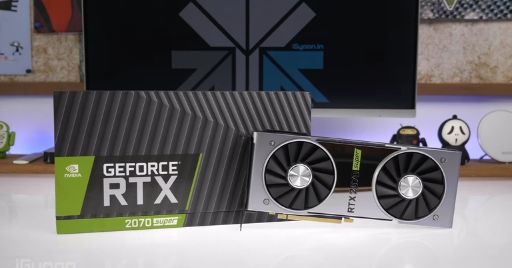
Fastest GPU For Deep Learning
SPECIFICATIONS
Brand: NVidia | Graphics Coprocessor: NVIDIA GeForce RTX 2070 | Graphics Manufacturer: NVidia | RAM Size: 8 GB | RAM Type: GDDR6 | Clock Speed: 1770 MHz
Here is the solution for those who don’t want to spend much but still want to get a GPU for deep learning. The NVidia GeForce RTX 2070 is one of the best GPUs for deep learning. After I have tested the GPU under different conditions, the results will be all.
Plus, I will also have this card compared with the previous 1070, so you will know whether it is worth the investment or not. First of all, let’s have a look at the specs. So, 2070 has 2,304 Cuda Cores, which are huge compared to the previous version, 1070, which had 1,920. Next, the base clock over 2070 is 1410; now, this is something that is limited here as compared to the previous 1070.
In terms of the boost clock, it is somehow similar to the earlier version and has no significant difference. However, everything set aside, the bandwidth over 2070 is somehow double compared to the previous 1070.
Also, the architecture I noticed here was Turing, not the pascal one, so you could imagine how well it would perform on algorithms. I also tried to play many games over this one at 4K was 38.4 points, while at 1440p, it was 64.2 points. However, for the sake of comparison, there was a gap between the 1070 and 2070, but I would not consider that huge.
While I tried another benchmark test, I discovered that 2070 cannot perform at 60 FPS at 1440p. But guys, if you compare the 3070 with 2070 for gaming, then the 3070 will definitely be the winner. If you are a ray tracing fan, then this GPU is pretty much decent at it, as we all know that 1070 didn’t have any hardware for that and literally was a flop at ray tracing.
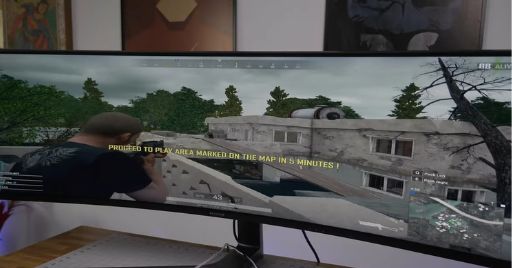
Now, let’s look at the power draw with 2070, so somehow I didn’t feel any difference compared to the previous 1070. In the end, I would say that if you want this GPU for gaming, just save some more money and get yourself a 3070 because it has better headroom for gaming as compared to any of the previous releases.
First, I was worried that the card would blow up because of the high temperature, but again thanks to its architecture, it remained cool all the time. The high temperature achieved was 73 degrees Celsius, but the fans were enough to cool down the system eventually.
Now I would not say this is the quietest GPU out there because it isn’t. This GPU does make noise, but it is not something that would bother you or disturb your roommate across the room. I was okay with the noise, but maybe some sound-sensitive folks might not be a fan of this one.
REASONS TO BUY
✓Remains cool
✓Decent gaming performance
✓Works for Ray tracing
✓Affordable price tag
REASONS TO AVOID
✗None, however, for some aesthetics might seem off.
WHY YOU SHOULD CHOOSE THIS GPU?
I believe NVidia GeForce RTX 2070 is one of the best graphics cards designed by NVidia for deep learning. You can get this one if you don’t want to upset your bank account; however, I would suggest not getting it for gaming as it is a deep learning processor. Other than aesthetics, there is not a single con about this GPU.
Conclusion
The profound learning revolution has finally arrived. AI-driven machines are taking over the world, and most of us can’t wait to start. But before we can build our AI assistants and Chabot’s, we must learn how to create them.
And while you don’t need a Ph.D. in computer science to do this, you’ll still need to choose the right GPU for deep learning. I have compiled some of the Best GPU For Deep Learning here.
Besides, we have provided some easy tips you can consider beforehand purchasing the best GPU for machine learning. Here are my favorites if you haven’t decided which one to get.
- If you want a powerful GPU for deep learning then I would recommend going for the NVIDIA Titan V, as it is one of the best powerful GPU for deep learning.
- NVIDIA GeForce RTX 2070 is the best budget GPU for deep learning if you don’t want to spend much
- And if you want to be extra and purchase the fastest GPU for deep learning then go for the NVIDIA TeslaV100.
So, are you ready to say hello to the future? Because we are! Order the best GPU for deep learning and get ready to experience the future!
Things To Consider For Buying Best GPU For Deep Learning
The best GPU for deep learning is a powerful graphics card that can handle complex mathematical operations quickly. Additionally, the card should be compatible with the deep learning software you will be using.
With the right card, you can have more fun while getting better results in your deep learning projects. This article provides a list of factors to consider when purchasing a GPU. There are a number of things you need to keep in mind when choosing the best GPU for deep learning. Here are some factors you should consider:
Power Requirements
The amount of power required by the graphics card should be taken into consideration when purchasing a GPU for deep learning. Most GPUs require an external power source, so it is important to make sure that your computer can support the additional power required.
If you plan on running several programs at once, you should also take into account the wattage needed by each program. The minimum wattage needed by a GPU is around 200 watts, but most modern computers have a maximum wattage rating of 250W or more. You should purchase a card that can handle the power requirements of your computer.
Compatibility
The compatibility of a GPU with deep learning software is another factor you should consider. Many programs that use GPUs can be run in the Windows operating system, but some must be run in a specific operating system.
For example, CUDA is popular software for deep learning. Some software can be run in multiple operating systems, so it is important to check compatibility before buying a GPU.
Price
A good GPU will cost more than a regular graphics card. However, there are some deals available that can lower the price considerably. Make sure to find the best deal when purchasing a GPU.
Performance
The performance of a GPU is another important factor to consider. The amount of memory the card has and its speed are key factors in determining how well a card performs. The higher the number of cores on a card, the better it performs.
When choosing a card, keep in mind that the number of cores is usually listed in the specifications section of the box. The higher the clock speed, the faster the card performs. Make sure that the performance of a card is enough for your needs. If you plan on running several programs at once, you should also take into account the wattage needed by each program.
Durability
The longevity of a GPU is an important factor to consider. Some cards are very fragile, so it is important to purchase a card that can handle the rigors of everyday use.
Features
Many graphics cards include additional features that can improve the performance of a card. The best graphics cards for deep learning include:
- Multiple types of memory. This includes GDDR5, GDDR3, and GDDR5X.
- DVI-D and HDMI ports.
- A large number of cores.
- High quality video outputs.
Cooling
A good GPU will require more cooling than a regular graphics card. The more powerful the card is, the more heat it produces. If you plan on overclocking your GPU, make sure that the card has adequate cooling.
Memory
The amount of memory on a card is an important factor in determining the performance of a card. The memory on a card can be divided into two types: RAM and VRAM. The amount of RAM on a card is measured in gigabytes (GB).
Most cards can handle up to 32GB of memory. RAM is used for general computing purposes and is not very important for deep learning. VRAM, however, is used for graphics, so it is vital for deep learning. VRAM is measured in megabytes (MB).
Some cards can hold up to 8GB of VRAM. This allows you to use more complex programs and take full advantage of the power of your GPU.
Cores
The number of cores on a card is another important factor when choosing a card. A card with a higher number of cores will usually perform better than a card with fewer cores. The more cores a card has, the more powerful it is.
If you plan on running several programs at once, the number of cores on a card is important. When choosing a card, make sure that the number of cores is listed in the specifications section of the box. A card with fewer cores may perform better if you only plan on running one program at a time.
Clock Speed
The speed at which a card runs is another important factor to consider. The clock speed is measured in megahertz (MHz).
The faster a card runs, the better it performs. When choosing a card, make sure that the clock speed is listed in the specifications section of the box.
If you plan on overclocking your card, make sure that the card can handle the rigors of overclocking. Some cards are designed to be overclocked, while others cannot be overclock.
Video Outputs
You should choose a card with a wide variety of video outputs. A card with only one or two outputs will not be able to display images and videos. Many cards include:
- DVI-D. This is the most common video output.
- HDMI. This is a high quality video output that allows for high resolution displays.
- DisplayPort. This is a high quality video output that can be used with high resolution displays.
- VGA. This is a standard video output that works with most monitors.
Frequently Asked Questions
What To Look For In A GPU For Deep Learning?
There are a few things you’ll want to look for in a GPU for deep learning. The first is horsepower; you’ll want a GPU that has a lot of horsepower. The second is memory bandwidth; you’ll want a GPU with a lot of memory bandwidth. And the third is power efficiency; you’ll want a GPU that consumes less power. Let’s go over each one.
How Much Would A GPU For Deep Learning Cost?
A high-end graphics processing unit (GPU) for deep learning could cost anywhere from a few hundred dollars to a thousand dollars. It all depends on what you want. There are also a lot of other things to consider, like the type of application you plan on using it for.
Do I Really Need A GPU For Deep Learning?
There is no definitive answer, as the performance of deep learning will vary depending on the specific application. However, a GPU can provide significant performance improvements, particularly when training complex neural networks.